If you’ve been reading this blog for a while, then, chances are, you’re probably testing. That’s good …
… at least some of the time.
Marketers who aren’t testing may actually be better off than the ones that are
If you’re not careful, you could be running tests that tell you one thing when, in fact, the situation is completely different. You could be making critical decisions based on bad data. And these are the worst decisions you could make, because you’ve got the data to confirm that you’re right, when you’re actually doing things incorrectly.
This is why we were so surprised when MarketingSherpa’s Landing Page Optimization Benchmark Report came out with the following chart in it:
–
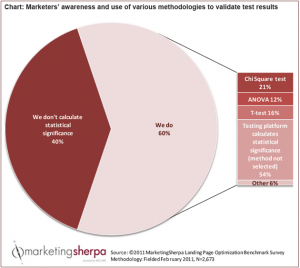
–
At least 40% of marketers who test don’t even account for statistical significance.
This means at least 40% of marketers who test are worse off than the ones that don’t test at all. Because, if you make business decisions based on unreliable data, not only could you make a bad choice, but you’ll have made that choice with the confidence of numbers. The surety of the data would have led you to this bad decision.
So how do we make sure our tests are telling the truth?
In a nutshell, we need to make sure they are valid. To do so, we must be able to identify and guard against four basic validity threats:
- Sample distortion effect
- History effect
- Instrumentation effect
- Selection effect
How do you identify and guard against these validity threats?
Well, here are two resources that should help you in your testing to guard against these four validity threats.
The first resource is to help you with the first validity threat … sample distortion effect. It’s a blog post to help you figure out when you have a large enough sample size for statistically significant test results. Daniel Burstein wrote it a while ago, and it was especially eye-opening . Here’s a link to the sample size blog post.
The second resource is a Web clinic replay titled Bad Data: The 3 validity threats that make your tests look conclusive (when they are deeply flawed) – educational funding provided by HubSpot. In it, Dr. Flint McGlaughlin walked us through the lesser known validity threats, the last three in my list above.
Hopefully these resources are helpful. And if you use other resources to guard against validity threats, feel free to share them in the comments section.
Related Resources:
Bad Data: The 3 validity threats that make your tests look conclusive (when they are deeply flawed) – Web clinic replay
Marketing Optimization: How to determine the proper sample size
Online Marketing Tests: How could you be so sure?
Email Optimization and Testing: 800,000 emails sent, 1 major lesson learned
–
It seems the web clinic replay was available for less than a week. The link for it is no longer valid.
@Alan Tutt
Alan,
Thank you so much for pointing that out. The link should be working now.